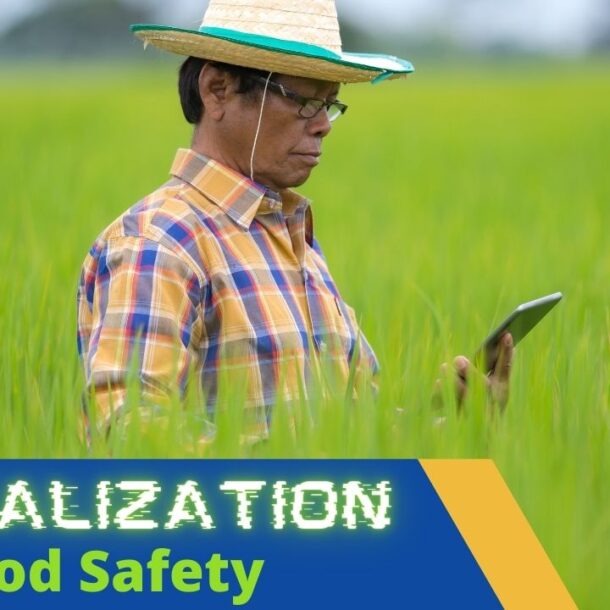
The predictive approach may not be per se a paradigm shift but most likely a complementary methodology to the preventive approach. For certain, we must shift our mindset to embrace it.
The predictive approach may not be per se a paradigm shift but most likely a complementary methodology to the preventive approach. For certain, we must shift our mindset to embrace it.
HACCP was born in the 1960’s to change a paradigm. After World War II, there was an increase in the production of food at large scale which was then distributed far away from its point of production. This brought new food safety challenges because at the time, the “quality control” reactive approach was commonplace in which product was tested after production. HACCP shifted this paradigm and introduced an effective preventive approach. Thus, we work to prevent contamination by identifying and assessing risks and hazards, defining GMPs, establishing prerequisites, defining and implementing standards and control measures, and so on. We conduct preventive measures to control hazards so they do not exceed acceptable levels in the end-product.
Nowadays, we have super computers, Artificial Intelligence (AI), digitalization and big data. Are we encountering a new paradigm shift? Can we move from a preventive to a predictive approach?
In recent years, we’ve heard a lot about new technologies and how they can change our lives for the better. We’ve learned a lot about blockchain and how it may positively impact the supply chain and increase trust along the food chain. Alongside blockchain, there has been another hype: predictive analytics.
Predictive analytics is the dream in which an application predicts what will happen in the future. What kind of scandal will we see? Which commodity will be contaminated? Where is food fraud happening?
To make a very long story short: it will never be this way. So, why even talk about it? Because there is no black and white, especially when it comes to data analysis and the real world. The strength of predictive analytics is that it can give you clues as to: Where you might see a scandal? Which commodity might be contaminated? Where food fraud might happen?
This is very important to understand. Everything in life comes with a probability. This statistical thinking is very different from the mechanical way of thinking learned in school. It represents the actual data (or truth) better because there is no 100% guarantee for anything. The mechanical way of thinking makes you just believe that. So, behind every analysis, there should be a measurement for accuracy, error, confidence, etc.
When we look at prediction, it is very natural, and we often do it every day. We just call it experience. When we wake up, we predict what we might do today. We try to predict how long we will need to drive to work, and we try to predict how the next audit will be. We then try to rely on our experience (what has worked so far) and gather new information (what will influence the outcome of our prediction). So, predictions do not necessarily have to use the latest machine learning algorithm but can start from using your experience and heuristics. Nevertheless, you get better insights and hints if you combine your experience with the use of advanced statistics and artificial intelligence. We see that this is often a journey, from guessing to stable, data driven insights and predictions.
If you are a quality manager and responsible to oversee your supply chain, here is how you can start to use predictive analytics: Start to visualize the non-conformities and shortcomings of your supply chain, no matter what the data source is. Connect to excel files, text files or other structured data sources. If you have your audit reports not in a structured way but in a PDF, it can make sense to use software like Adobe Acrobat to export information into an excel file. This is more work for sure, but this will jumpstart your data journey. Then look for patterns in your data in a BI tool like Excel, PowerBI or Tableau. A pattern that often occurs is the occurrence of higher numbers of the same NCs in a supply chain, region, or commodity. Discuss your outcomes with your peers and coworkers to get a good understanding of the validity of your insights, quality of your data and other, possible patterns to look for. Then you can use this insight to plan your following audits. In general, you have five questions to answer, what we call the “risk-based audit question”: Whom to audit when, how, what shall be the content of the audit and who shall perform the audit. Based on your flagged insights you may then come up with a list like this: John Doe witness audits the Tasty Cooky Bakery next month and the focus will be on CCPs. The next step then can then be to use some external data besides your audit data. The RASFF database for example can give you good hints what to look for in your supply chain. Use such signals and integrate it when you answer the risk-based audit question.
One of the greatest advantages of digitalization is how much easier it is to analyze and share data. If you work in a big company with multiple sites in the same country or even across the globe you can join efforts with your peers to use all the data to build up better prediction models. Even if you work in a SME, don’t be discouraged by it and consider building cooperation across companies to gather information. It may feel overwhelming at first, so start with one company that is easier to invite to join you (you may have a friend who works there with whom your boss has a good relationship) build from there.
This is the “manual” way of predicting and it is a good start. It gives you first insights. The challenge is, that we as humans are not very good at recognizing more complex patterns. Humans can work with a maximum average of three to four variables at the same time. Audit data has much more complexity. This is the bottle neck when we look for patterns like inconsistencies, similarities etc. manually. Computers and algorithms are exactly made for this. They can store almost a limitless number of variables and patterns and compare them to each other. Nowadays, this is called data mining these days. After you have validated and tested the outcomes of this approach, you then store these insights in a so called “model”. From this point on, whenever you prepare for a new audit or you want to look for patterns in auditees or auditors, you can use this model to highlight the weak parts and biases that may occur.
Another feature is your model can learn over time. You can retrain these models when new data comes in. So, improvement continues over time. This allows you to arrive at better answers to the risk-based audit questions.
The predictive approach may not be per se a paradigm shift but most likely a complementary methodology to the preventive approach. For certain, we must shift our mindset to embrace it.
We must look to all the data produced by our Food Safety Management System (FSMS) not as an end-all but as the beginning. Sometimes it seems that FSMS are built to generate data. They aren’t! The data produced by a FSMS should be the starting point for its improvement but also as a source of information we can build on to predict what we should focus on more the next time we conduct an audit or assessment, for example.
Developing a collaborative approach (and mindset) in the food industry to collect and use data is as challenging as it is necessary if we want to embrace this new HACCP frontier.
This article was written with Jochen Kleboth, head of Analytics and AI at Intact.
Intact works with compliance data. This means Intact provide a software tool for standard setters, certification bodies and producers to gather, process and analyze data from first-, second- and third-party audits. This software tool allows us to help our customers find inconsistencies in their data, analyze root causes and the ability to predict non-conformities. Intact helps customers understand which checklist points are worthy to look at in greater detail and which checklist points are more likely to be acceptable. For example, it could be that there is a pattern of having a wrong CCP for a certain processing step repeatedly for a certain kind of food business operator. This particular insight may be used to plan supplier audits with greater focus or use this insight to trigger e.g., an unannounced audit. Furthermore, if a supplier audit is significantly different from what you predicted, then this is worth looking at to identify potential inconsistencies and food safety risks. This is a simple example which demonstrates the power of using data driven predictions and automatic insights.
Disclaimer: The information contained on this article is based on research done in the last months and the authors personal experience and opinion. It is not intended to represent the view of any organization they work for or collaborate with. The authors will not be held liable for the use or misuse of the information provided in the article.